Financial risk prediction has been the focus of fintech.
Recent years have witnessed the rapid popularization of internet finance (e.g., internet insurance, peer-to-peer (P2P) lending, and consumer finance) in response to the demand for new financial services with higher efficiency and convenience. This raises again, in the new context of fintech, the core issue of financial risk management. Generally, financial risks cause damage to various stakeholders and may even weaken the stability and sustainability of the overall economy. Accordingly, financial risk prediction (FRP), as an important instrument for taking precautions against losses due to financial risks, has been a focus of fintech for decades. It is expected that FRP will be able to reveal potential financial risks in the near future, thus providing early warning signals and supporting related decision-making.
Research status regarding FRP
There are two levels for FRP in terms of financial entities: default risk prediction (DRP) corresponding to individual lenders and financial distress prediction (FDP) corresponding to listed companies. And the existing regarding DRP and FDP incorporates two aspects. In terms of the features, both DRP and FDP studies have long relied predominantly on a single information source, leading to homogeneous features and the incomplete evaluation of financial risks. In terms of methodology, the statistical methods and machine learning methods have been more widely used in FDP. Moreover, there is now a trend of using ensemble methods to improve prediction performance over single classifiers in both FDP. Nowadays, with the increasing popularity of online platforms and social media is resulting in more and more heterogeneous sources of predictive information, including both hard information and soft information, hence introducing new possibilities. By leveraging the characteristics of big data, such as high variety and high volume, learning from multisource heterogeneous soft information has the potential to lead to further improvements in performance.
Challenges in DRP when the heterogeneous information is involved for FRP
However, existing FRP methods designed to deal with homogeneous predictive information sources are not easily adaptable to new scenarios with multisource heterogeneous information. Such scenarios present some new challenges, such as heterogeneous feature sources, heterogeneous feature structures, heterogeneous feature implications, and feature interactions. Since certain feature sources or feature structures naturally have their own unique predictive abilities, indiscriminately superposing or simply selecting multisource features may not fully capture their potential and may even hurt prediction performance. This new phenomenon calls for a mechanism that can make effective use of valuable information available from heterogeneous sources by appropriately tackling the new challenges they pose.
We introduce a novel hybrid-strategy-based self-adaptive method for FRP
|
|
|
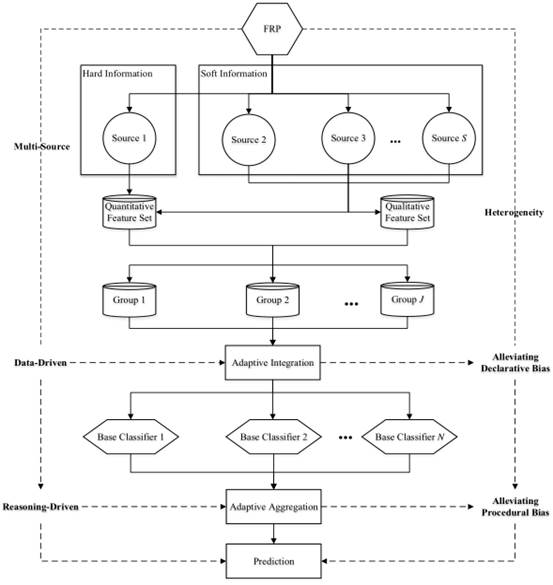 |
We strive to develop a new FRP method that can effectively leverage multisource information with heterogeneous structures. We extract multiple types of features with heterogeneous structures based on data from a rich set of information sources and make multiple methodological contributions at different levels. We propose a novel hybrid-strategy-based self-adaptive method, named HSB_RS (for hybrid-strategy-based random subspace), which adaptively integrates the multisource heterogeneous features and adaptively aggregates the predictions of a set of diverse base classifiers, thereby alleviating both the declarative bias and the procedural bias of the learning process. Our HSB_RS fundamentally adopts the framework of random subspace (RS) and further leverages the strengths of two new methods we propose specifically within HSB_RS. One is a new feature-sparsity learning method called WFAL_GW (i.e., weight-fused adaptive lasso incorporating group weighting) for adaptively integrating multisource heterogeneous features. The other is an improved evidential reasoning (ER) rule instantiated in and tailored to the context of classification called AER
(i.e., adaptive ER) for adaptively aggregating the predictions of diverse base classifiers.
Figure 1. Framework for a Hybrid-Strategy-Based Self-Adaptive Method
Our main contributions
This study makes several contributions to the literature. First, it contributes to the design science paradigm of IS by proposing a novel artifact, a hybrid-strategy-based self-adaptive method (HSB_RS) for FRP to better leverage heterogeneous multisource data that are becoming increasingly prevalent. By adaptively integrating heterogeneous multisource features and adaptively aggregating the predictions of multiple base classifiers through two proposed algorithms (i.e., WFAL_GW and AER), HSB_RS alleviates both the declarative bias and the procedural bias in a machine learning process. Second, this study provides a general method for predicting financial risks at different levels, e.g., FDP at the company level and DRP at the individual level. Such unification provides inspiration for researchers to enrich their methodologies toward broader applicability. Third, this study takes into account multisource heterogeneous information, including both hard information and soft information. It empirically demonstrates the usefulness of some emerging sources of data, thus broadening our knowledge about useful predictors for financial risks.
The managerial implications
Our proposed method presents a new way for practitioners to achieve improved risk predictions by leveraging a variety of data sources. It may be further applied to emerging phenomena, e.g., predicting financial risks associated with the recently flourishing P2P lending platforms themselves (besides borrowers), which has practical implications on the investment decisions of lenders. It may even be adapted to other practical contexts, e.g., financial fraud detection (Abbasi et al 2012) and customer churn prediction.
Information
Wang Gang, Chen Gang, Zhao Huimin, Zhang Feng, Yang Shanlin, Lu Tian. Leveraging Multi-source Heterogeneous Data For Financial Risk Prediction: A Novel Hybrid-Strategy-based Self-Adaptive Method. MIS Quarterly, 2021, 45(4): 1949-1998.